Understanding the role of "racist algorithms" in AI ethics discourse
Includes a bonus recipe for a self-licking ice cream cone
On Saturday, March 27, Kareem Carr unwittingly stepped on a very famous Twitter landmine. The Harvard doctoral student in statistics posted the following thread, and when I saw it in my feed I knew even before he did that he was about to need a medevac:

Carrโs thread made substantially the same points that got Facebook AI chief Yan LeCun driven off Twitter temporarily this past summer by an AI ethics rage mob. In fact, I saw Carrโs thread via LeCunโs approving retweet of it.
In my own thread in reply, I predicted that Carr would get grief for this, and what the response from the AI ethics crew would be. Former Google AI ethicist Margaret Mitchell was the first to respond according to the exact script Iโd already laid out, but there were others over the course of the weekend.

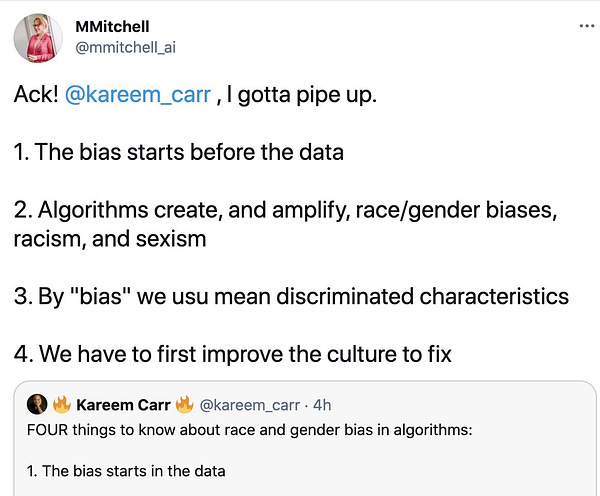

The following 48 hours were filled with tweets and subtweets, and tweets-then-deletes, and lengthy explainers. But despite the mounting pressure, Carr didnโt budge โ he didnโt apologize, nor did he retract the thread. I cheered him on from my own account because, despite our many differences, I could see that Carr was both meaningfully correct and as aware of (and appalled by) the tactics being deployed against him as I was.
The weekend drama with Kareem Carr is a window into the very heart of the entire AI ethics project. The core question that Carr tried to tackle โ the question of โwhat does it mean to allege that an algorithm has race or gender bias?โ โ is the one question the AI ethicists are most keen to answer in a very particular way. But their way is at odds with Carrโs way.
It is important to understand why Carrโs thread had to be opposed so vigorously, and what work the claims of โalgorithmic biasโ are doing in the discussions around fairness, accountability, and transparency in machine learning.
In short, the โalgorithmic biasโ claims are a key ingredient in a self-licking ice cream cone of doom. Hereโs how it works.
Iโm so sorry, but we gotta talk CRT for a sec
To understand the opposition to Carrโs thread, we have to talk about Critical Race Theory (CRT). I know... I know... critical race theory (CRT) is the all-purpose right-wing boogeyperson that always gets the blame for everything wrong in the world, but in this case, you really canโt grasp why Carrโs ultimate claim โ that bias problems are โfixableโ โ riled up a whole section of AI/ML twitter without some context.
I think the best compact summary of CRT, at least for my present purposes, comes from one of its most prominent critics in aย recent interview:
Critical Race Theory is an academic discipline that holds that the United States is a nation founded on white supremacy and oppression, and that these forces are still at the root of our society. Critical Race Theorists believe that American institutions (such as the Constitution and legal system), preach freedom and equality, but are mere โcamouflagesโ for naked racial domination. They believe that racism is a constant, universal condition: it simply becomes more subtle, sophisticated, and insidious over the course of history. In simple terms, critical race theory reformulates the old Marxist dichotomy of oppressor and oppressed, replacing the class categories of bourgeoisie and proletariat with the identity categories of White and Black. But the basic conclusions are the same: in order to liberate man, society must be fundamentally transformed through moral, economic, and political revolution.
Despite the fact that it comes from a detractor, this thumbnail sketch squares with the academic CRT Iโve read, and more importantly, it accurately sums up the way that this discourse manifests on Twitter. Indeed, I have a hard time imagining a supporter of CRT disagreeing with any of the above (though Iโm always open to being corrected).
So in the eyes of CRT, racism is:
The most important part of the origin of every (interlocking) system in our society โ government, markets, education, religion, military, etc.
Still present and pervasive in all those systems, and indeed it actively structures them.
Not possible to separate from any of those systems while leaving them intact in anything like their present form. A complete overhaul of all the interlocking parts is needed.
The above framework applies not just to CRT, but to critical theories generically โ you just swap out (or complement) โracismโ with other -isms, -phobias, and prefixed flavors of patriarchy.
That last point โ that you canโt just separate out a problematic -ism from systems and institutions, and fix it in isolation, leaving everything else intact โ is key, because itโs at the root of the objections to Carrโs claim that bias in AI is โfixable.โ
The self-licking ice cream cone recipe
One of the reasons the three-part critical theory framework above is so pervasive in different fields and disciplines, is that it provides a universal template for producing arguments, papers, conference speeches, and other artifacts of scholarship in any area.
In short, critical theory is a recipe for turning any professional or scholarly domain into aย self-licking ice cream cone, where the goal is to keep everyone busy with pursuing a goal that can never be reached.
The recipe for producing critical theory work is as follows:
Take as a starting point that we all agree on some flavor of the three fundamentals of critical theory I laid out above.
Explain, ideally via some novel set of connections or moves that no one has seen before, how racism (or sexism, or cissexism, or cisheteropatriarchy, etc.) actively structures your object of study.
Call for the object of study to be โdismantledโ or otherwise completely reimagined, and rebuilt in some ism-free fashion so that it no longer perpetuates harm.
Optional: Give some concrete, detailed ideas for what should replace the thing you called for the reform (or even abolition) of in the previous step.
Again, that third step is the most important โ a โgoodโ critical theory product (i.e., a paper, a Twitter thread, video, etc.) is one that systematically andย convincingly removes any possibility of a targeted, in-situ fix for the object of criticism, leaving either total overhaul or outright abolition as the only way forward.
(Itโs actually the case that in the news mediaโs output, that third, activist step is typically omitted. The reportโs job is done when they point out how hopelessly broken the system is, and they leave the calls for reform or abolition to others.)
Anything that smacks of incrementalism or technocratic tweaking will be rejected. The object of criticism must be dismantled with all haste โ not patched and left in production, but pulled offline entirely and rebuilt. To suggest otherwise is to be complicit in the harm the object is causing.
You can do this sort of work with everything fromย British gardening, toย prairie dresses, toย the Snyder Cut, toย early American history, to machine learning. The algorithm works with any object, and if youโre really good at producing novel scholarly artifacts with this mechanism, then you can make a career out of it.
Here's an example of the recipe at work in a recent paper,ย Towards decolonising computational sciences.
Secondly, we explain how centering individual people, as opposed to tackling systemic obstacles, is a myopic modus operandi and indeed part of the way the current hegemony maintains itself. Fundamental change is only possible by promoting work that dismantles structural inequalities and erodes systemic power asymmetries...
Computational and cognitive sciences โ fields that both rely on computational methods to carry out research as well as engage in research of computation itself โ are built on a foundation of racism, sexism, colonialism, Anglo- and Euro-centrism, white supremacy, and all intersections thereof (Crenshaw 1990; Lugones 2016). This is dramatically apparent when one examines the history of fields such as genetics, statistics, psychology, etc., which were historic- ally engaged in refining and enacting eugenics...
The system itself needs to be rethought โ scholars should not, as a norm, need to form grassroots initiatives to instigate retractions and clean up the literature. Rather, the onus should fall on those pro- ducing, editing, reviewing, and funding (pseudo)scientific work. Strict and clear peer review guidelines, for example, provide a means to filter racist pseudoscience out (Boyd, Lindo, Weeks, & McLemore 2020). Ultimately, it is the peer review and publishing system, and the broader academic ecosystem that need to be re-examined and reimagined in a manner that explicitly excludes harmful pseudoscience and subtly repackaged white supremacist from its model...
Individual level issues such as interpersonal displays of racism are not the cause but a side-effect, symptomatic of a much deeper problem: structural, systemic, social, and insti- tutional racism and sexism โ ideals and values set in place purposefully a couple of centuries ago (Saini 2019).
I couldโve just quoted the whole paper โ itโs all along these lines on every page.
Model bias in AI Ethics
There were two diagrams posted in response to Carrโs thread that illustrate the above beautifully. The first is from a tweet by Deb Raji:
This is quite a good diagram of the multi-step process of building and deploying a machine learning model. I really like it. The red labels denote touch points where the humans who perform these steps โ working as they do in a context of privileges, -isms, and -phobias โ can taint the process and the outcome with their biases.
Itโs important to be clear on Rajiโs goal in presenting this diagram labeled in this way: she is not aiming to highlight sites where this system can or even should be fixed or improved. Rather, the goal is to show that bias is pervasive at every step, with the result that the system in the diagram is not fixable.
The only solution is to work backward from those red labels and reform the larger context that causes humans to transmit their biases via these touchpoints.


To repeat for emphasis: every red-labeled type of bias in that diagram is alleged to beย immune to a technical fixย (though some mitigations are admitted to be possible). The only way forward is aย complete transformationย of our society.
A related flowchart was posted in reply to Rajiโs thread by feminist Paolo Bonomo, interestingly enough as a retweet from where she had originally posted it in the context of the LeCun vs. Gebru fight over this same issue in the summer of 2020.
Yet again, the point of this diagram is that there is the possibility of humans introducing their biases at every step, so there can be no technical fix. Theย bias structures the entire social system in which the technology is embedded, and cannot be eliminated without transforming society.
Unpacking algorithmic bias
Hereโs an important fact about Deb Rajiโs diagram that I think Raji and her colleagues understand quite well, but that I think is not so widely appreciated outside the world of machine learning:ย all of the red-labeled human touchpoints in the top half of the diagram can and will be eliminated by some flavor of self-supervised learning (SSL) in the near future.
At some point soon, the only types of โAI biasโ left for the critical theorists to problematize, will be the two types associated with the model itself: aggregation bias and evaluation bias. Thatโs it. (More on SSL in a future post.)
The additional, post-output types of bias identified in that second diagram are all part of the human context in which AI systems are embedded, so theyโll continue to get attention from critical theorists. But for various reasons Iโll cover in a moment, this isnโt satisfactory โ the critics want to keep working on โAIโ proper.
In most AI ethics contexts (especially on Twitter), both types of bias in the bottom half of that diagram are collapsed into โalgorithmic biasโ or โmodel bias.โ When the claim is made that the algorithms or models themselves are biased, they mean the parts of the diagram labeled โaggregation biasโ and โevaluation bias.โ
Itโs actually refreshing to see this โmodel biasโ argument broken up into two parts, because normally these things are both conflated and then gestured at, and the people doing the gesturing typically donโt seem to realize theyโre talking about multiple things.
I have not Googled either of these terms, so I donโt know the specific backstory on how Raji is using them here, but Iโve read so many AI ethics papers I know exactly what goes in the boxes those terms are labeling:
In the spot labeledย aggregation bias, there are alleged biases that go into selecting the model architecture and the training algorithm. This is the math-intensive part that the alpha nerds are paid big money for. (In AI ethics lore, these alpha nerds are white males. In real life, they are indeed mostly males, but in my experience and that of ML people Iโve interviewed, the dominant identity categories are Asian, white, and Jewish.)
Where her diagram hasย evaluation bias, there are alleged biases that go into the construction of metrics (also a math-heavy enterprise) that are used to determine if the model is performing as intended.
I say โalleged biases,โ because this is where all the game-playing and shenanigans happen. This is where it gets good.
Number games
Go back to our critical theory recipe, zoom in on step 2 (i.e., โExplain... how racism... actively structures your object of studyโ) to get to Deb Rajiโs diagram, then zoom in on that one โModelโ box in the middle with all the math in it. Youโre now looking at the critical, load-bearing pillar that is supporting the main wing of the AI ethics edifice.
That box full of math has a number of properties that make it an ideal object for critical inquiry:
Itโs aย black boxย in the classic sense, in that itโs making decisions that the people downstream from it have no insight into or control over.
The math inside it isย advanced and gate-keeperyย โ itโs understood by relatively few, and produced by even fewer. So itโs suspect on that score.
The people who make that mathย all tend to look and act a certain way, at least in the AI ethics mythology. (No one to my knowledge has ever done a survey of the ethnic makeup of elite ML research teams, however. And certainly, no one has done an attitudes survey of them to see if they conform to popular techbro stereotypes. These claims about the makeup of the field are anchored in anecdote and premised on the notion that Asians of all varieties are functionally โwhiteโ in an ML research context.)
As I pointed out above, itโll beย the only human-touched box left standingย in the realm of ML proper when SSL finally eats the whole field.
Now Iโll walk you through a toy example of the alchemy that lets an AI ethicist turn a bit of math into โracism.โ
Hereโs a collection of ten balls of three different colors. Our task is to represent this whole collection as one ball of a single color. What color do we choose?
If we average the colors weighted by the number of balls, then the average ball color in this collection is a shade of gray. That lone gray ball, then, becomes representative of the entire collection despite the fact that itโs the only one in the group that looks the way it does.
If we select the color thatโs represented in the collection more than any other, then weโll end up with a white ball standing in for the whole group, despite the fact that the white balls make up just half the group.
If those balls were people and the colors were skin colors, then the black people in this group would get erased under either scheme. That is algorithmic bias.
Now, I didnโt say โthat is algorithmic bias, in a nutshell.โ Because thereโs no nutshell. This is literally how every single paper, tweet, video, and talk works, just with harder math.
If youโre a sane person, you no doubt find it hard to credit my claim that this is all thatโs going on. Nonetheless, it is true. I wrote a whole essay on this, and if you want the technical details you can jump to the appendix. Every example of โalgorithmic biasโ I was able to find reduces to: โthis model or statistical technique suppressed one set of data points and/or amplified another set,โ where the data points in question map to some human characteristics.
For instance, hereโs this exact logic at work in a tweet from a Google Brain researcher and professor, but done with curve-fitting math:


Itโs always the same point, everywhere all the time in every paper from every person who writes about this:
The math under investigation amplifies one corner of the dataset and/or suppresses another.
The corner of the data that got short shrift at the end corresponds to some marginalized identity out in the world.
Ergo, the model itself introduces racist bias. QED
You can do the above work โ i.e., show that a piece of math amplifies/suppresses signals that correspond to human racial categories โ in both of the red-labeled boxes in Deb Rajiโs diagram:
You can select a model definition that, when paired with a particular dataset thatโs encoded in a particular way, suppresses signals that happen to correspond to minorities in the real world.
You can select a set of evaluation methods that either donโt address #1 above or make it worse by motivating you to tweak the model in the wrong direction.
There is stats-type math in both of these boxes, and it does that amplification/suppression thing Iโve been on about for the whole life of this newsletter.
All of this results in a situation where every time a researcher sticks a new bit of math in one of these boxes, there is a chance that an implementation engineer will run some human-derived data through it and find that the model is โbiasingโ the results along race/gender/etc. lines. And whenever this happens, a conference paper can be written about that problem and added to the literature on โalgorithmic bias,โ where it will eventually make its way into tweets, talks, and books about how algorithms themselves can be inherently racist independent of the input data.
Unpacking the boxes further
Iโve made all the points I want to make about bias, so if youโre tired of reading about this you can skip this section and not miss much. But for completenessโs sake, I want to return to the ball example and break it down in terms the AI ethics people use.
Bias in data collection and labeling: The ball collection in my image is my data, and you can see that it has a certain makeup that directly lends itself to the problems Iโm having compressing it or otherwise representing it in a way thatโs โfair.โ If the balls were colored differently, or there was a different number, the outcome would be different.
Bias in the problem statement: I characterized the task as โrepresent the whole collection as one ball of a single color.โ The way the task is framed means Iโm definitely going to erase one or more colors, no matter what my data looks like. Maybe my results would be more equitable if the task where, say, โrepresent the collection as a ball filled in by a gradient.โ
Bias in the algorithm: My approach of using either the most common color or a weighted average of colors doomed me to erase the black balls in the output.
Bias in evaluation: What if Iโm looking at the results with my diversity, equity, and inclusion hat on? With that hat on, I believe the collection would be best represented by a ball color that has historically been underrepresented. Itโs important that we redress this historical inequity, so if the algorithm is producing white and gray balls, then I have to keep sending it back for tweaking until it shows me a black ball.
You can easily imagine how I could go on doing this with this toy ball problem. I could probably squeeze another six or seven types of bias out of it. So it shouldnโt be hard to envision how a group of highly educated and motivated people could mine a complex, math-heavy, fast-moving field like machine learning for an endless amount of this type of material.
This is especially true if youโre not in any way constrained by a requirement that you propose a technical fix for any problems you identify. Because the problems are unfixable, and indeed proposing a fix is anathema, the only constraint on the production of critical theory artifacts are institutional โ how many papers can we publish and talk about, how many jobs are there for doing this work, who can I get to notice and signal boost the problematizing Iโm doing, and so on.
The self-licking ice cream cone has a cyanide in it
Anyone who has followed my Twitter feed or this newsletter knows Iโm in the camp that believes AI/ML has real problems, and real potential for widespread harm. The AI ethics folks have at times pointed out real issues that demand urgent attention, and I credit them with bringing these things to light in a way that has caused many of us to begin focusing on them. I certainly would not be aware of or interested in these issues if it werenโt for their work.
But where I part company with them is the same place Kareem Carr does โ with โitโs fixable.โ Iโm in the โmend it, donโt end itโ camp, and I think the way forward is to continue to work on mitigations, fixes, and tweaks to the system we have.

One of the fixes that will have to be deployed if weโre to make progress, is to dismantle the self-licking ice cream cone. Whatโs needed is a counter-revolution in AI/ML โ some kind of fairness, accountability, and transparency ML (FATML) caucus thatโs dedicated to making things better, and that values rigor, fairness, and civility.
This will require people within AI/ML who are willing to openly confront the existing FATML clique, and argue with them about both substance and style. Because leaving the problem of fixing ML to revolutionaries whose project is to put the goalposts so far down the field that they can continue playing the game indefinitely is a recipe for rolling catastrophe.
I find this article extra disappointing because you're consistently so close. I'll work through this with a concrete example, because I think that makes things simpler.
You seem to agree with 1-4 from your society has problems tweet, yet you seem to take issue with people suggesting that the solutions to those problems can't be entirely technical, and that in some cases the cost of perpetuating society's problems today may be greater than the value gained from automating them.
Say we propose to replace cash bail with a risk model, as California recently voted on. There are lots of possible models one could propose. Trivially, you could hold everyone, or let everyone free. Both of those present statistical bias: the model will misclassify some people. But does the model have any racial bias? Well a model that lets everyone free arguably does not (and you're free to argue this, please do!), but a model that holds everyone perpetuates any existing biases in the police force, so if for a particular group is more likely to be arrested even if they aren't more likely to commit crime, they'll be held in jail more than they should be. A statistical *and* racial bias.
But note that if the police force isn't racially biased to begin with, the (naive, but still) statistically biased algorithm won't make things worse.
But we don't use those naive algorithms, we use complex ML models. And in those cases, they can encode additional bias not solely present in the initial data. Other people and papers explain this far better than I can, but imagine you overpolice a specific group, the data will show that they're more likely to commit crime. If you train a model on that data, you now have the police arresting people more, and the model holding more of those people, a double dip of racial bias, despite only a single-dip of statistical bias.
So we both start from the assumption that "society has problems", then one possible goal is to reduce the statistical bias as close to zero as possible. This, at best, makes things no worse than they are. But even a perfectly statistically unbiased algorithm may increase inequality, if for example its use makes some pre-existing socially biased system more efficient.
So the question then is "what are you mending?" Reducing statistical bias is usually, probably, a good thing. But not always: sometimes intentional biases in a system reduce inequality although this can be controversial along multiple axes. For a non-ML example, consider voting districts gerrymandered to be majority-minority so that minorities would have some representation. This requires thinking about intended outcomes: should congress (or the bag of marbles) represent the mean citizen or the median? Which is more important: geographic or racial representation?
"Better data" or "better algorithms" won't solve those problems. And naively applying ML, even "unbiased" ML may exacerbate those problems. You can similarly see this issue in debates around algorithmic approaches to redistricting, and how those may result in less-representative districts in some cases, even if they do reduce partisan gerrymandering.
Further, you seem to take serious issue with many of the ethicists, framing them as rather radical for saying that we should be cautious about how we approach using ML and similar tools. But their position is a fundamentally conservative one. If, right now, at this very moment, ML isn't being used somewhere, it is more radical to add some sort of ML than to change nothing.
Here, you claim that the ethicists don't propose solutions beyond toppling the whole system (where the whole system may not just mean ML, but all of society or something). But that isn't true. In googles-colosseum, you acknowledge that there's another solution they propose: intentionally modifying the data (or the model) to better represent the way the world "should" be. You claim that this is a non-starter for Google, but it isn't. They absolutely do it in some cases already. Perhaps it isn't possible in all cases, for technical reasons, but then the question of "should we introduce a system that perpetuates inequality that we all agree is there" is a really important one! Maybe the value is still worth it, but maybe it isn't, so we should ask the question (and some of the work of the ethicists you criticize is frameworks for addressing similar questions).
On twitter, you also seemed to have a personal aversion to the "Separation" approach. If I understood your argument correctly, it was that since we can't all agree on the way we want the world to be, the best approach is to not try to influence things, and just have the models do what they do (https://twitter.com/jonst0kes/status/1374827973677834246).
There's a number of problems with this though. First of all, someone's gonna need to make choices that impact the system. Something as simple as the L1 vs. L2 norm can affect how a model perpetuates inequality. So ultimately it isn't HRC vs. SBC, it's HRC or SBC or the Google engineers building the model. Someone is evaluating its performance. You can't escape that.
And you might say that your response, that the Google engineers don't represent "activists with a niche, minority viewpoint", which might be true. But that doesn't mean that the model represents the linguistic status quo. Just the opposite in many cases: there's lots of cargo-culting of various meta-parameters (e.g. lists of topics or words that should be excluded when building the model) that perpetuate inequality (and statistical bias). Even if you consider that good work, and it's only a subset of the work by the activists that's objectionable, it's still not clear that what you'll end up with. Are you really sure that a model built by a company with the ultimate goal of being profit-driven will be better for you representative than one who aim for it to be socially responsible? Also, I think you almost stumble on one of the major criticisms of LLMs but carefully avoid it: what if you could have both the HRC and the SBC model, and could pick between them? Then the issue is entirely political: you convincing Google which one to use (or offering both). As is, it's cost prohibitive to build both
Back to the main point: the thesis of many of the ethicists is that technical fixes to a model won't fix everything. That's very different than how you represent them, which appears to be that they are anti-technical fixes. Beyond that, you claim that they offer no solutions. This is half true: they are careful to say that there's no silver bullet. But they do offer tools to improve things, both technical and organizational/social/procedural. You seem to reject many of those non-technical tools, not because they're fundamentally, but because of personal aversions to the politics of the people offering them. But framing them as radical end-its, when they're ultimately conservative-in-how-we-apply-and-use-these-tools people who propose solutions you're personally averse to seems profoundly unfair.
There's also a meta-conversation that continued focus on only the technical fixes is counterproductive to solving the (again: real problem that we all agree on) that ML will amplify inequality in some cases. Fixing the bias doesn't fix the inequality. So saying the bias is fixable won't fix "it", where "it" is what pretty much every lay person cares about.
incisive, clear thought and timely observations most welcome in this time of madness. A+, tweeting all about it, as I said, a banger.